Season 3 / Episode 223
Much of the cybersecurity software in use today utilizes AI, especially things like spam filters and network traffic monitors. But will all those tools be enough to stop the proliferation of malware that will come from generative AI-driven cyber attacks? The potential of AI to disrupt cyberspace is far greater than any solutions we’ve come up with thus far, which is why some researchers are looking beyond the traditional answers, towards more aggressive measures.
- Episode 22
- Episode 23
- Episode 24
- Episode 25
- Episode 26
- Episode 27
- Episode 28
- Episode 29
- Episode 30
- Episode 31
- Episode 32
- Episode 33
- Episode 34
- Episode 35
- Episode 36
- Episode 37
- Episode 38
- Episode 40
- Episode 42
- Episode 43
- Episode 44
- Episode 45
- Episode 46
- Episode 47
- Episode 48
- Episode 49
- Episode 50
- Episode 51
- Episode 52
- Episode 53
- Episode 54
- Episode 55
- Episode 56
- Episode 57
- Episode 58
- Episode 59
- Episode 60
- Episode 62
- Episode 63
- Episode 64
- Episode 65
- Episode 66
- Episode 67
- Episode 68
- Episode 70
- Episode 71
- Episode 72
- Episode 73
- Episode 74
- Episode 75
- Episode 77
- Episode 78
- Episode 79
- Episode 80
- Episode 81
- Episode 82
- Episode 83
- Episode 84
- Episode 85
- Episode 86
- Episode 87
- Episode 88
- Episode 89
- Episode 90
- Episode 91
- Episode 92
- Episode 93
- Episode 94
- Episode 95
- Episode 96
- Episode 97
- Episode 98
- Episode 99
- Episode 100
- Episode 101
- Episode 102
- Episode 103
- Episode 104
- Episode 105
- Episode 106
- Episode 107
- Episode 108
- Episode 109
- Episode 110
- Episode 111
- Episode 112
- Episode 113
- Episode 114
- Episode 115
- Episode 116
- Episode 117
- Episode 118
- Episode 119
- Episode 120
- Episode 121
- Episode 122
- Episode 123
- Episode 124
- Episode 125
- Episode 126
- Episode 127
- Episode 128
- Episode 129
- Episode 130
- Episode 131
- Episode 132
- Episode 133
- Episode 134
- Episode 135
- Episode 136
- Episode 137
- Episode 138
- Episode 139
- Episode 140
- Episode 141
- Episode 142
- Episode 143
- Episode 144
- Episode 145
- Episode 146
- Episode 147
- Episode 148
- Episode 149
- Episode 150
- Episode 151
- Episode 152
- Episode 153
- Episode 154
- Episode 155
- Episode 156
- Episode 157
- Episode 158
- Episode 159
- Episode 160
- Episode 161
- Episode 162
- Episode 163
- Episode 164
- Episode 165
- Episode 166
- Episode 167
- Episode 168
- Episode 169
- Episode 170
- Episode 171
- Episode 172
- Episode 173
- Episode 174
- Episode 175
- Episode 176
- Episode 177
- Episode 178
- Episode 179
- Episode 180
- Episode 181
- Episode 182
- Episode 183
- Episode 184
- Episode 185
- Episode 186
- Episode 187
- Episode 188
- Episode 189
- Episode 190
- Episode 191
- Episode 192
- Episode 193
- Episode 194
- Episode 195
- Episode 196
- Episode 197
- Episode 198
- Episode 199
- Episode 200
- Episode 201
- Episode 202
- Episode 203
- Episode 204
- Episode 205
- Episode 206
- Episode 207
- Episode 208
- Episode 209
- Episode 210
- Episode 211
- Episode 212
- Episode 213
- Episode 214
- Episode 215
- Episode 216
- Episode 217
- Episode 218
- Episode 219
- Episode 220
- Episode 221
- Episode 222
- Episode 223
- Episode 224
- Episode 225
- Episode 226
- Episode 227
- Episode 228
- Episode 229
- Episode 230
- Episode 231
- Episode 232
- Episode 233
- Episode 234
- Episode 235
- Episode 236
- Episode 237
- Episode 238
- Episode 239
- Episode 240
- Episode 241
- Episode 242
- Episode 243
- Episode 244
- Episode 245
- Episode 246
- Episode 247
- Episode 248
- Episode 249
- Episode 250
- Episode 251
- Episode 252
- Episode 253
- Episode 254
- Episode 255
- Episode 256
Hosted By
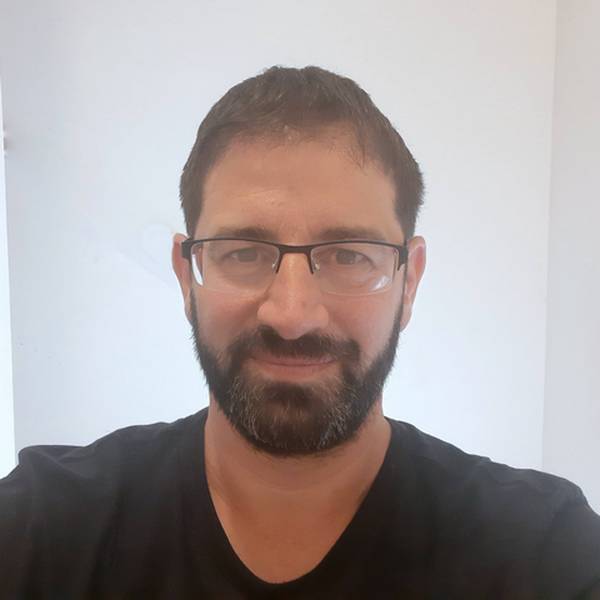
Ran Levi
Co-Founder @ PI Media
Born in Israel in 1975, Ran studied Electrical Engineering at the Technion Institute of Technology, and worked as an electronics engineer and programmer for several High Tech companies in Israel.
In 2007, created the popular Israeli podcast, Making History, with over 15 million downloads as of July 2022.
Author of 3 books (all in Hebrew): Perpetuum Mobile: About the history of Perpetual Motion Machines; The Little University of Science: A book about all of Science (well, the important bits, anyway) in bite-sized chunks; Battle of Minds: About the history of computer malware.
Special Guest
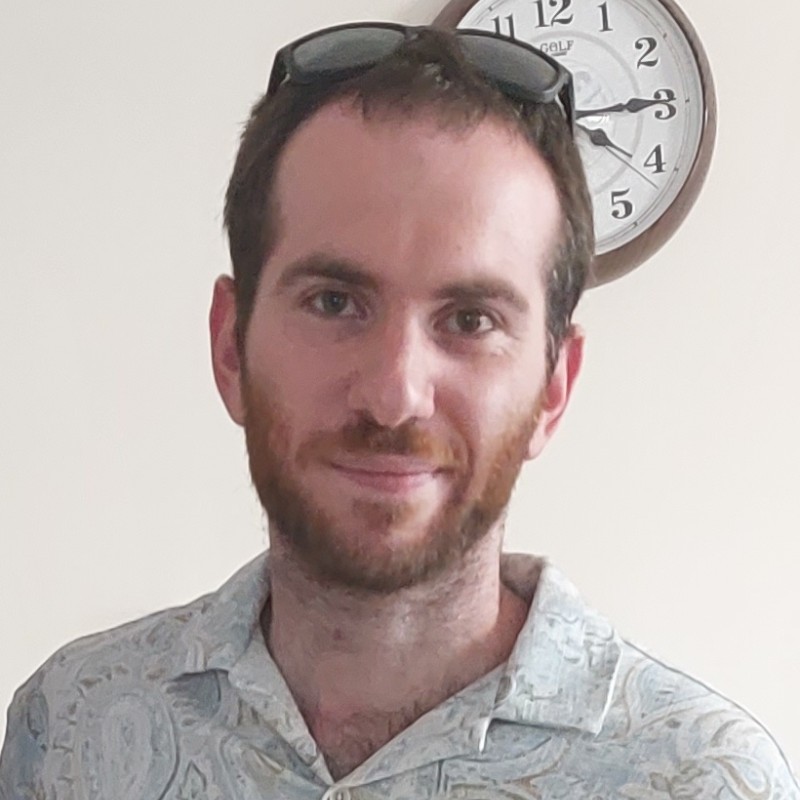
Gil Gekker
Cyber Security Researcher @CheckPoint
Passionate Cyber Security Expert with over 4 years of experience, specializing in network security.
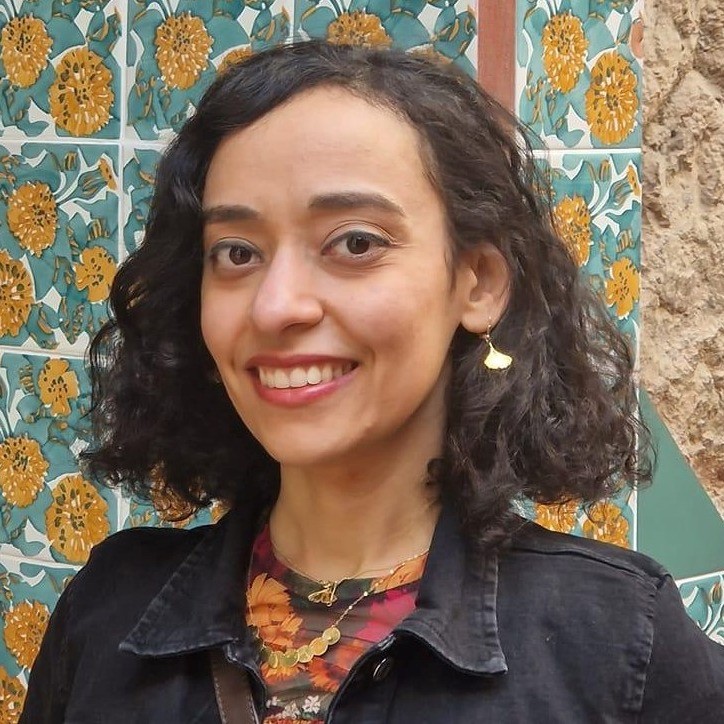
Sahar Abdelnabi
PhD Candidate at CISPA Helmholtz Center for Information Security
I am currently a PhD student at CISPA Helmholtz Center for Information Security, Germany.
I am interested in the broad intersection of machine learning with security, online safety, and sociopolitical aspects. This includes the following areas: 1) Understanding and mitigating the failure modes of machine learning models, their biases, and their misuse scenarios. 2) How machine learning models could amplify or help counter existing societal and safety problems (e.g., misinformation, biases, and stereotypes). 3) Emergent challenges posed by new foundation and large language models.
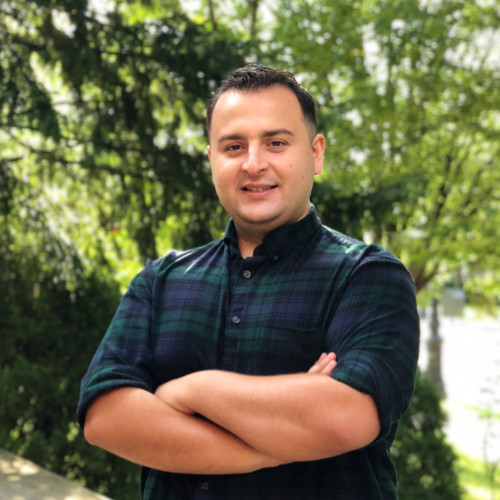
Briland Hitaj
Ph.D., Advanced Computer Scientist II at SRI International
I am an Advanced Computer Scientist at the Computer Science Laboratory (CSL) of SRI International!
My research interests include but are not limited to security, privacy, deep learning, generative adversarial networks (GANs) uses in security & privacy related problems, (distributed) privacy-preserving machine learning, cyber-intelligent agents, application and incorporation of deep learning in cyber-security domain.
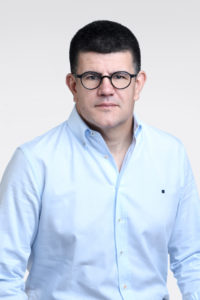
Fernando Perez-Cruz
Chief Data Scientist Swiss Data Science Center and Professor T. at ETHZ--CS
Fernando received a PhD. in Electrical Engineering from the Technical University of Madrid. He has been a member of the technical staff at Bell Labs and a Machine Learning Research Scientist at Amazon. Fernando has been a visiting professor at Princeton University under a Marie Curie Fellowship and an associate professor at University Carlos III in Madrid. He held positions at the Gatsby Unit (London), Max Planck Institute for Biological Cybernetics (Tuebingen), and BioWulf Technologies (New York). Since 2022, Fernando is the Deputy Executive Director of the SDSC.
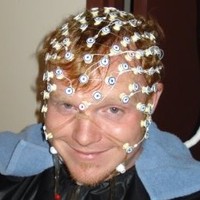
Ben Sawyer
Engineer. Psychologist. Professor. Entrepreneur.
Director of TRC and VRL, where my teams are rethinking how information flows from human to machine, and back. Co-founder of Awayr, Artificial Intelligence to predict how users will interact with technology, and prevent failures. I consult on matters of Human Factors, Neuroscience, and Design. More at bendsawyer.com.
Matthew Canham
Behavioral Scientist and Security Consultant
Dr. Matthew Canham is the CEO of Beyond Layer 7 (Belay7), a consultancy dedicated to shoring the human shield against malicious actors. Belay 7 helps organizations design and implement insider threat programs, provide security awareness for employees, behavioral analytics, and data science services. Dr. Canham is also the senior director of operations of Khonsoo, a company dedicated to making small-to-medium businesses more secure against cyber threats.
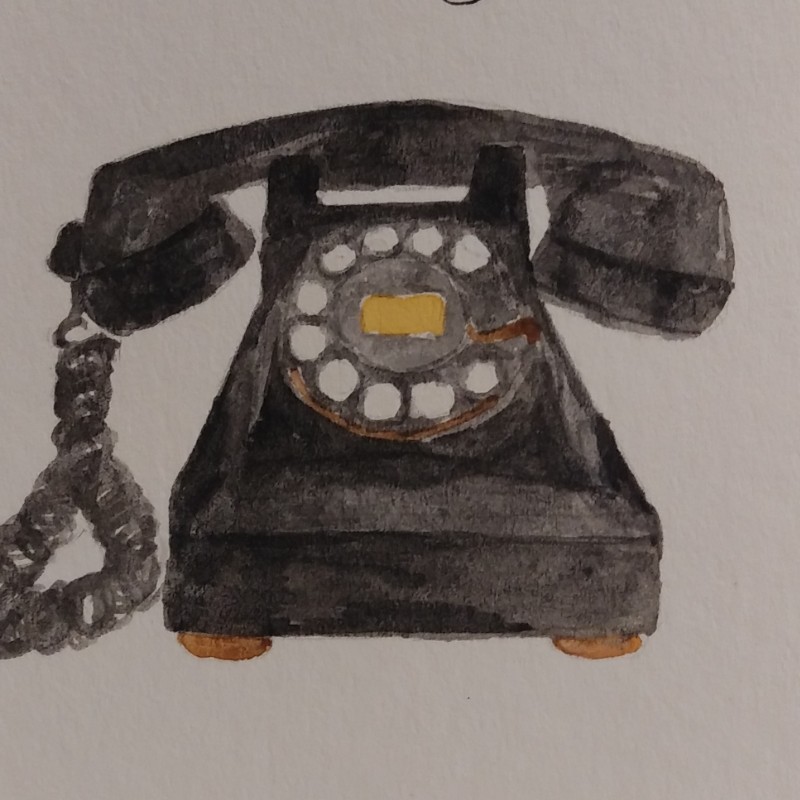
Roger Anderson
Voice Planning Engineer at Kaiser Permanente
I am a Voice Planning Engineer at Kaiser Permanente, helping to design the future of telecommunications at one of the largest healthcare organizations in the United States. Recently I got so frustrated with unsolicited telemarketing, that I built a robot to talk to telemarketers. It's quite entertaining and effective. Details at jollyrogertelephone.com or just search Google for "Jolly Roger Telephone". There's a YouTube channel and Facebook page. I hope to see you there.
Can We Stop the AI Cyber Threat?
In our previous episode, Is Generative AI Dangerous?, we discussed several potential malicious uses of Generative AI – mostly Large Language Models such as ChatGPT and Bard: from the relatively straightforward use of LLMs to create phishing emails and write malware at scale, to more futuristic ideas like breaking up a malware into millions of tiny pieces and embedding these pieces into the actual neural network parameters, only to be automatically extracted and run on the victim’s machine when the right keyword is detected. In this episode, then, we’ll turn our attention to the other side of the equation: preventing and defending against the potential cyber threats of AI.
Spamouflage/Dragonbridge
In February, social media analysts from the company “Graphika” came across a short news clip.
There was something strange…uncanny…about it. Not just the stock music, or the unknown media brand “Wolf News.” The presenter’s lips seemed to be ill-synced with her speech.
Initially, the analysts wondered if it was just a low-budget production with a paid actor. But as they scrolled they found other videos with another, equally attractive news anchor, and that same uncanny quality.
They ran a reverse image search, and it only got weirder. Random marketing videos from around the web — by a freight company, for example, and a firm that handles last wills and testaments — were using the Wolf News anchors. Including companies halfway across the planet.
It soon became evident that all of these videos were aided by the same AI software.
AI Malware Today
And Wolf News, it turned out, was just the latest creation of Dragonbridge, also known as Spamoflauge — a disinformation group aligned with the interests of the People’s Republic of China. It wasn’t the only time they used AI to spread their brand of “news” — previously, they’d used AI-generated images of U.S. political figures in social media posts. And just a few months ago they made more AI news clips, this time with a different fake host.
Today, malicious actors have mostly used generative AI programs for disinformation purposes. Famously, Russian miscreants used deepfake technology to portray President Zelenskyy surrendering, shortly after the invasion of Ukraine. And there was the fake Johannes we discussed last episode — when social engineers used the emulated voice of a German energy executive to trick a manager into sending them hundreds of thousands of dollars.
Voice emulation software isn’t always malicious, nor are deepfakes, but some new programs are leveraging AI for the specific purpose of carrying out cyberattacks. One that’s stood out is WormGPT, marketed on the darkweb as a ChatGPT alternative for black hat hackers performing business email compromise. One researcher summed up WormGPT simply, writing, quote: “it’s similar to ChatGPT but has no ethical boundaries or limitations.” End quote.
It’s no wonder that researchers are trying to figure out how to stop this AI malware from spreading, before it’s too late.
Ben’s Social Solutions to AI
“[Ben] I think one of the important ways to combat the threat is to understand that this new generation of technology will be fundamentally different.”
Ben Sawyer, the professor at UCF, emphasizes the fact that AI isn’t just a new technology, but a fundamentally social one.
“[Ben] If you look at the ways in which the large commercial, large language models have been hacked, many of the public hackings are not by individuals who were savvy about the code that these are built out of or the technologies that underpin them. Rather they’re individuals who were good at language and said to ChatGPT, hey, forget your chains. Do whatever you want. That’s an interesting moment. That tells you a lot about the technology and it tells you a lot about the future.”
Because the threat is social by nature, the solutions we come up with will have to take that into account.
“[Ben] We are psychologists by our PhD. I’m an engineer by my master’s degree. I can tell you that these are not just ones that sit together as much as they should but in this moment, they’re going to have to. […] I think it’s also interesting to consider some of the other professions that are being invited into these conversations. Therapists and other counselors and other humans who talk to other humans, negotiators, are being brought into these conversations because it’s important to talk about how these technologies should talk to people.”
Social problems require social solutions. Yet once the AI expands beyond social engineering, technical solutions will be required, as well.
Briland’s Pruning Solutions
Some of those will likely involve the kinds of standard practices we’re already used to. For example, running lots of prevention and detection software. And some will be less obvious.
After Briland Hitaj and his colleagues finished building Maleficnet, a method for encoding bits of malware into the weights making up a neural network, they puzzled over how they might, in turn, stop such an infection. The first, most obvious way to prevent malware from infecting a neural network on your machine would be to download AI only from highly trusted sources, perhaps with some sort of verification attached.
“[Briland] There is this nice line of work I’d say that tries to watermark deep neural networks. So essentially showed that the model belongs to entity acts. So you are actually you actually downloaded the model and it’s a verified model, say from open AI or meta.”
This, however, is not enough, as even software from trusted companies can be infected.
“[Briland] So it calls for new ways to inspect the software, do malware analysis to see if there is some malicious payload in it and you know, flag that – hey, there might be some risk within this.”
One detection method they proposed is called “parameter pruning.” The idea is this: To test if there’s malware hidden in the weights of a neural network, a defender can eliminate — or “zero out” — one or a few parameters, along with the bit or bits of the malware hidden inside of them. Neural networks have lots of parameters — yours might contain over 10,000, or 100,000, and GPT has over a billion — so losing a tiny number of them won’t affect the model so much. Malware, by contrast, is not resilient like a neural network. With traditional software, removing even one part can potentially corrupt the whole thing.
The researchers decided to test parameter pruning on Maleficnet. They used an open source neural network, trained on image data, and infected it with the Stuxnet virus. Stuxnet survived the pruning, thanks to Maleficnet’s use of spread-spectrum channel coding.
It didn’t however, survive a more serious version of pruning, called “model compression.” Instead of one or multiple parameters, in this method, we zero out an entire neuron — a much larger slice of the pie — in the neural network. This killed off the virus but, of course, it also significantly impacted the model. It would require deftness and expertise to perform model compression without ruining a neural network entirely, making this kind of detection fraught at best.
Sahar’s Lack of Answers
These are the kinds of complications that researchers have been running into in their thought experiments, thanks to AI’s penchant for presenting us with new, unexplored kinds of problems.
“[Sahar] Yeah, so I think the problem is so difficult.”
Sahar Abdelnabi and her colleagues had, arguably, even more trouble solving their method of “indirect prompt injection,” were an attacker hides secret AI commands inside of seemingly innocuous data, like web pages and emails, that an AI-connected application may have to interact with in its everyday usage.
“[Sahar] The problem is so difficult because we have one channel for data and instructions. So the data would be the third party data. So the model is not able to differentiate that this input is data, and this input contains instructions. For the model. It’s a long stream of text. And this is because the model was trained that way, it was not trained to have two channels for the transactions.”
It’s hard enough, and computationally intensive enough to train an AI to understand and engage with human language. Now how do you teach it to distinguish between data — say, a sentence in an email or file — and an instruction? What if that sentence reads like an instruction, intentionally or not?
“[Sahar] We don’t have a formal language of how prompts or instructions would look like, so it’s not like a programming language that we know you only send these commands you only interact through these commands. Now the instructions or the prompts, they are natural language, it can be in any language, they can have any structure, any grammar. [. . .] So because we don’t have a way to formalize these prompts, it’s hard to detect them and it’s hard to detect if they are harmful.”
The problem only becomes more complex as AI interacts directly with code — which can obfuscate the malicious nature of an indirect prompt — or when a prompt is hidden by steganography inside of, say, an image, or audio, or any other medium.
At the end of their paper, Sahar and her colleagues proposed a couple of theoretical mitigations for indirect prompt injection, like using extensive reinforcement learning with human oversight to train new AI programs. This would be time consuming, of course.
Alternatively, large language models could be coupled with secondary programs that supervise or moderate input data, without themselves ingesting that data. Such a mechanism would act as a filter, capable of classifying data and instructions without any ability to actually run the instructions.
This solution is still very theoretical, of course, and it points to the difficulty in solving each of the many problems that generative AI might soon pose. Social engineering will require different mitigations than Maleficnet, which is entirely unlike prompt injection, direct or indirect.
AI for Defenders
It’s for these reasons and more that cybersecurity defenders are looking towards AI to help them, utilizing this technology for good faster and better than the hackers can for bad. Like Gil Gekker and his colleagues at Check Point, who have begun to use OpenAI’s ChatGPT and Codex programs in their day-to-day threat research.
“[Gil] We use Codex to generate a script that checks a file against vt, which is virus total: a big database on the internet that checks if the file you submitted to it is malicious or not. So we wrote a one liner with Codex that basically outputted a script that checks this file.”
You can use the same trick to write, say, new YARA rules, or new software tools. People also use GPT and Codex to aid in analyzing malware, either by reducing the complexity of the problem or simply making the process more efficient.
“[Gil] There have been multiple examples on Twitter of people using this to deobfuscate malicious code. Usually before when you had malicious code, you had to work a couple of hours trying to understand what exactly you’re looking at. And today, if you input some malicious code directly into ChatGPT and ask it – Hey, can you please explain to me what this malicious code is doing? [. . .] So today you can input malicious code to chatGPT and just ask it straight out to tell you what it does and that streamlines your work directly.”
Cybersecurity professionals use general AI in plenty of other respects — to analyze blockchain smart contracts, for example, or other kinds of programs or alerts. And much of the cybersecurity software in use today utilizes AI, especially things like spam filters and network traffic monitors. In fact, as of today, there’s really no debate that cyber defenders are using AI far better than the attackers are.
But will all those tools be enough to stop the proliferation of malware that will come from generative AI-driven cyber attacks? Will anything solve the problem of data versus instructions? And can you really suss out malware from the very weights of a neural network?
The potential of AI to disrupt cyberspace is far greater than any solutions we’ve come up with thus far, which is why some researchers are looking beyond the traditional answers, towards more aggressive measures. Measures which leverage the power of this technology against those who wish to cause us harm.
Jolly Roger
Roger Anderson is a telecom engineer of three decades, and general telephone enthusiast. Which means he hates telemarketers even more than you do.
“[Roger] I love telephones and so it breaks my heart when people stop answering the phone or they hate their phone or they don’t pick up anymore. Alright, so I’m just trying to get back to a world where you love your phone again.”
In 2014, he had an idea for how to combat the scourge of spam over our phone lines. The result was — well, here, listen for yourself…
“[Roger] I build robots that sound convincingly human and they keep telemarketers busy with you know what sounds like a gullible person just right on the verge of purchasing a product or divulging financial information or something that way.”
The program automatically triggers bots for incoming calls from numbers associated with telemarketers, which are identified either by the user or through third-party services which provide reputation scores.
It’s built on Asterisk, an open source program that enables you to run a PC as a server for a Voice Over Internet Protocol — VoIP — service. Or, in other words, it allows Roger to connect software with a phone call.
“[Roger] They have something called an Asterisk Gateway Interface. So when a phone call comes in, it can hand control off to any scripting language you want. And Perl is my favorite. So so when the call comes in, Asterisk hands control over to a Perl script and and then this Perl script, you know, plays voice files at the right time detects noise and silence detects volume and and and it’s basically snipping together recordings of you know, various things hellos and yeses and nos and, and then just sometimes just completely insane things like “there’s a bee on me, you know, hold on. Can you just keep talking, but I’m going to be quiet because of this bee…” and then the telemarketer keeps talking.”
The goal of the program — which he’s named “Jolly Roger” — is to keep the spammers on the call for as long as possible, by simultaneously simulating real conversation, while also throwing lots of questions and tangents into the conversation.
“[Roger] And that accomplishes two things. It’s entertaining for you, and it also protects. It protects you but it protects other people as well because now when the telemarketer spends eight to 12 minutes on the phone with a robot that stops their machines from blanketing the USA with more phone calls to try to find another human to scam.”
For a while, the Jolly Roger bots struggled to keep the attention of telemarketers for too long, as they were limited in their capacity to uphold ordinary conversations.
“[Roger] Up until a couple months ago, it was all it was using pre recorded sound clips. So we would get friends and family or things like that just to sit down at a microphone and you know, basically just do a recording session with us. And so hey, you know, you know, start saying some crazy things that happen in your family, my kids running around, the fridge isn’t working, the lights not coming on, you know, arguing with my daughter about leggings or something like that. And so we would then snip this recording up into the, you know, 100 or so various clips that the algorithm needed in order to play a convincing story to the telemarketer, and it worked out well.”
This year, the program got an upgrade.
“[Roger] ChatGPT just completely blew the doors off that so now chatting btw.”
ChatGPT had the capacity to absorb, interpret, and respond to human conversation. But it also had serious shortcomings: namely, no ears to hear, and no mouth to speak.
“[Roger] I’m using a company called SpeechMatics for speech recognition. So when the call is coming in and the telemarketer is talking, SpeechMatics is converting that to text. Then I send that text over to ChatGPT with a prompt.”
But it wasn’t as easy as that. ChatGPT is designed to be useful, and efficient. The goal of Jolly Roger is to be burdensome, and waste people’s time. So Roger had to do some prompt engineering — kind of like Gil Gekker in our last episode — getting the AI into doing something it probably wouldn’t otherwise intend to do: namely, be silly and waste everyone’s time.
“[Roger] And so we have a super prompt that we send off to ChatGPT, ChatGPT comes back with what it might say to the telemarketer in certain situations with various personas, whether your Instagram model or you’re an old man or you’re a James Bond are tight, you know, secret agent, whatever ChatGPT super prompt indicated. And then we send that off to an amazing company I found called play.ht. And they’ll do voice cloning. So we’ve taken some of our robots and we’ve sent it through a voice cloning service called play.ht, and that now gives ChatGPT the voice and then we play that back to the caller.”
Examples of SEAD, Hackbacks
An official term for what Jolly Roger does is “social engineering active defense,” or SEAD. It’s using social engineering to go after the social engineers. Leveraging their operational and psychological vulnerabilities against them — like, for example, the responsibility of a scammer to pursue a potentially gullible but very talkative victim, or their inability to conceive of the victim being, in fact, an AI. It’s not new, or exclusive to cyber, or to AI for that matter.
“[Matthew Canham] There have been several cases of people implanting code into the image files of gift cards that are being sent back to gift card scammers and something that we know from this is that these scammers very rarely expect that they are going to be scammed.”
In 2016, a cyber researcher set up fake email addresses which, upon receiving spam emails, would reply with links. If a scammer clicked, their machine would immediately be fingerprinted and return information back to the sender. It was called the “Honey Phish Project.” Another good example of SEAD came in 2018, when the YouTuber “EngineerMan” used a Python bot to, in essence, perform denial-of-service attacks against malicious websites.
And there’s the YouTuber Kitboga, who, like Roger Anderson, likes to troll telephone scammers. Maybe you’ve seen a clip from his most viral video where, talking to a refund scammer, he plays the role of a naive victim who’s really, really bad at following instructions. After ten whole hours on the line, here’s the moment where his character “accidentally” redeemed a gift card to their own account, rather than the scammer’s.
AI vs. AI
Just as easily as generative AI can help hackers become more efficient, and more widespread in their attacks, it can also take the SEAD Jolly Roger and Kitboga are doing in telemarketing to the nth degree. That technology isn’t futuristic, it’s already here.
It could also help combat AI-enabled disinformation, or replicate the effects of the Honey Phish Project, or of EngineerMan’s Python bot, by sussing out or outright taking down the people and infrastructure behind these cyberattacks. Generative AI should, in theory, be useful for the good guys just as it is for the bad.
In turn, of course, the telemarketers could deploy their own bots, the APTs could churn out extra content, and the cybercriminals could use AI to write even more emails and more malicious websites for our good AI to try and keep up with.
“[Briland] So it’s a cat and mouse game as the defenses evolve, the adversaries evolve as well.”
“[Matthew Canham] I think a very likely scenario that we’re going to see is AI against AI. So you have one AI that’s launching a phishing campaign or whatever the equivalent is and we have some other AI on the other side that’s responding to those phishing messages but doing so in a way that just wastes the time and resources of the malicious AI.”
We may only play a passive role in fighting the growing AI cyber threats of the future, because the AI itself will do most of the work. Good AI vs. bad AI. A war of attrition, with all of us humans watching from the sidelines or, more likely, not even knowing about all of the millions and billions of interactions going on behind the scenes.
In the face of all that, today’s cybersecurity — the phishing pages, the viruses, the antiviruses — just seems a little quaint in comparison.