Season 3 / Episode 104
There are plenty of reasons why Police should use AI for facial recognition: after all, Humans are notoriously bad eye witnesses. However, placing AI in the hands of law enforcement does have its dangers - due to the limitations of the technology itself, and the biases of the officers who use it.
- Episode 22
- Episode 23
- Episode 24
- Episode 25
- Episode 26
- Episode 27
- Episode 28
- Episode 29
- Episode 30
- Episode 31
- Episode 32
- Episode 33
- Episode 34
- Episode 35
- Episode 36
- Episode 37
- Episode 38
- Episode 40
- Episode 42
- Episode 43
- Episode 44
- Episode 45
- Episode 46
- Episode 47
- Episode 48
- Episode 49
- Episode 50
- Episode 51
- Episode 52
- Episode 53
- Episode 54
- Episode 55
- Episode 56
- Episode 57
- Episode 58
- Episode 59
- Episode 60
- Episode 62
- Episode 63
- Episode 64
- Episode 65
- Episode 66
- Episode 67
- Episode 68
- Episode 70
- Episode 71
- Episode 72
- Episode 73
- Episode 74
- Episode 75
- Episode 77
- Episode 78
- Episode 79
- Episode 80
- Episode 81
- Episode 82
- Episode 83
- Episode 84
- Episode 85
- Episode 86
- Episode 87
- Episode 88
- Episode 89
- Episode 90
- Episode 91
- Episode 92
- Episode 93
- Episode 94
- Episode 95
- Episode 96
- Episode 97
- Episode 98
- Episode 99
- Episode 100
- Episode 101
- Episode 102
- Episode 103
- Episode 104
- Episode 105
- Episode 106
- Episode 107
- Episode 108
- Episode 109
- Episode 110
- Episode 111
- Episode 112
- Episode 113
- Episode 114
- Episode 115
- Episode 116
- Episode 117
- Episode 118
- Episode 119
- Episode 120
- Episode 121
- Episode 122
- Episode 123
- Episode 124
- Episode 125
- Episode 126
- Episode 127
- Episode 128
- Episode 129
- Episode 130
- Episode 131
- Episode 132
- Episode 133
- Episode 134
- Episode 135
- Episode 136
- Episode 137
- Episode 138
- Episode 139
- Episode 140
- Episode 141
- Episode 142
- Episode 143
- Episode 144
- Episode 145
- Episode 146
- Episode 147
- Episode 148
- Episode 149
- Episode 150
- Episode 151
- Episode 152
- Episode 153
- Episode 154
- Episode 155
- Episode 156
- Episode 157
- Episode 158
- Episode 159
- Episode 160
- Episode 161
- Episode 162
- Episode 163
- Episode 164
- Episode 165
- Episode 166
- Episode 167
- Episode 168
- Episode 169
- Episode 170
- Episode 171
- Episode 172
- Episode 173
- Episode 174
- Episode 175
- Episode 176
- Episode 177
- Episode 178
- Episode 179
- Episode 180
- Episode 181
- Episode 182
- Episode 183
- Episode 184
- Episode 185
- Episode 186
- Episode 187
- Episode 188
- Episode 189
- Episode 190
- Episode 191
- Episode 192
- Episode 193
- Episode 194
- Episode 195
- Episode 196
- Episode 197
- Episode 198
- Episode 199
- Episode 200
- Episode 201
- Episode 202
- Episode 203
- Episode 204
- Episode 205
- Episode 206
- Episode 207
- Episode 208
- Episode 209
- Episode 210
- Episode 211
- Episode 212
- Episode 213
- Episode 214
- Episode 215
- Episode 216
- Episode 217
- Episode 218
- Episode 219
- Episode 220
- Episode 221
- Episode 222
- Episode 223
- Episode 224
- Episode 225
- Episode 226
- Episode 227
- Episode 228
- Episode 229
- Episode 230
- Episode 231
- Episode 232
- Episode 233
- Episode 234
- Episode 235
- Episode 236
- Episode 237
- Episode 238
- Episode 239
Hosted By
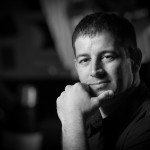
Ran Levi
Born in Israel in 1975, Ran studied Electrical Engineering at the Technion Institute of Technology, and worked as an electronics engineer and programmer for several High Tech companies in Israel.
In 2007, created the popular Israeli podcast, Making History, with over 14 million downloads as of Oct. 2019.
Author of 3 books (all in Hebrew): Perpetuum Mobile: About the history of Perpetual Motion Machines; The Little University of Science: A book about all of Science (well, the important bits, anyway) in bite-sized chunks; Battle of Minds: About the history of computer malware.
Special Guest
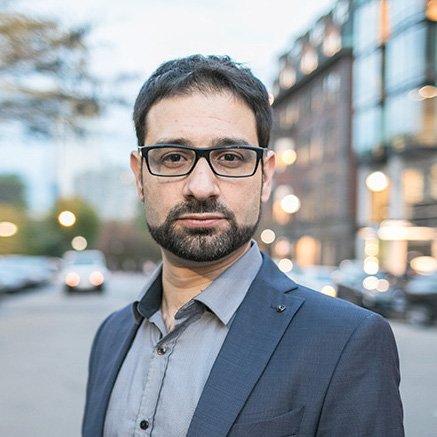
Yossi Naar
Chief visionary officer,co-founder at Cybereason
Co-Founder of Cybereason - a leader in innovative solutions for Malop protection and EDR (Endpoint Detection and Response) solutions. I have many years of experience developing specialized security solutions for defense contractors. I am also a skilled software architect and product manager. During my career i built many products ranging from embedded low-level systems through desktop application to complex big data systems.
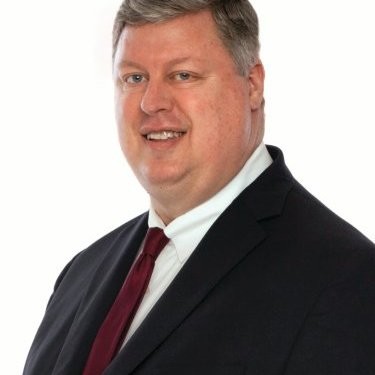
Ted Claypoole
Partner at Womble Bond Dickinson (US) LLP
I'm a lawyer in Atlanta, Georgia, on the FinTech Team, the Privacy and Cybersecurity Team and leading the IP Transaction Team at Womble Bond Dickinson. For more than 30 years, I have represented clients in regard to software and service agreements, data management including privacy/security/ analytics, payment systems, and technology planning. My work for current firm clients includes major financial institutions, colleges and universities, manufacturers and distributors, data security companies, software firms, hospitals and health systems, governments and government contractors.
Should Law Enforcement Use Facial Recognition? Pt. 1
859 Florida-50, Clermont. Wednesday. 5:10 P.M.
The Hilltop Ace Hardware store in Clermont, Florida is just about the last place you’d expect to be host to a groundbreaking case of grand theft.
It’s a boxy, one-story building with mulch stacked up just about everywhere, flanked by a gas station and a flooring store. Inside it’s a typical hardware store, you know how they are–cramped, with all kinds of tools and things lining every inch of shelf space. It’s tough to imagine that anyone would come to a place like this for anything other than, like, a new screwdriver.
But on the evening of November 20th, 2019, two people walked in with greater ambitions. One of them was a white woman–rugged features, brunette hair pulled back into a ponytail, dressed in a black pencil dress and flip-flops. With her was an African-American man dressed much better for the job–sunglasses, and very indistinct single-colored t-shirt, pants and hat. Also flip-flops. (Do all Florida criminals wear flip-flops? That doesn’t seem like the greatest idea.)
The two drove up to Clermont’s Ace Hardware, backing their black pickup truck into a parking spot directly in front of the store. They went in and grabbed, among other things, two grills and a vacuum. Pretty unexciting stuff, but by the time they drove off they had $12,000 of stolen merchandise in that pickup.
Police obtained security footage, and ran some of the images through a facial recognition algorithm. Simple enough, happens all the time. What made this case unique was that the software used by Clermont police didn’t actually identify the woman by her face.
It used a particular camera shot which caught her whole body, and used it to focus on an entirely different body part. In the shot, you can see a little mark on the woman’s left ankle–a tattoo. A standard facial recognition algorithm wouldn’t care about such a thing. But in 2019, Clermont happened to be trialing a new software called “Clearview AI.”
Clearview uses not only government and police records but also billions of social media pictures to feed its algorithm. That’s what allowed it to pick out 31-year-old Heather Reynolds who, in addition to looking like the suspect, had the same tattoo in her Facebook pictures.
FACIAL REC USED TO SUCK
Hi and welcome to Cybereaon’s Malicious Life Podcast. I’m Ran Levi.
Facial recognition wasn’t always ankle-tattoo-good.
“[Ted] As with many of these technologies, it was a stair step process.”
Ted Claypoole is a lawyer, and an author on legal issues surrounding privacy and AI.
“[Ted] through the aughts, facial recognition existed but it wasn’t great until about 2015. It wasn’t something that would really be usable whereas the FBI of course has a – as long had a biometric database of fingerprints for example. But their face print database really wasn’t great until recently and then the clearview database which takes all of the social media pictures into account has really multiplied the abilities to use this kind of database.”
Before Clearview, police departments around Florida relied on a program called “FACES”–the “Facial Analysis Comparison and Examination System.” Based on an algorithm developed by the French company Idemia, it works by cross-referencing a database of Floridians’ mugshots, driver’s licenses, as well as digital photographs taken by police officers.
But FACES just isn’t that great. According to the New York Times, Florida officers queried the system as much as 4,600 times a month at its peak, but only a small fraction yielded anything resembling results. It took four years for the system to aid in an actual arrest and, according to the New York Times, in its 20 years of operation only around 2,000 successful arrests have been causally tied to FACES.
That number doesn’t include questionable cases, or accurate identifications which didn’t lead to arrests, and it’s worth noting that it took awhile for FACES to spread statewide. But still: 2,000 arrests is not a lot for a place as big as Florida. It’s even less impressive when you consider just how expensive the software is. FACES was built off a 3.5 million dollar government grant in the year 2000 and, by 2014, ended up eating 14 million in taxpayer dollars.
So that’s 14 million dollars, divided by 2,000 arrests…let’s see…drag the one and…
Yep: 7,000 dollars per arrest. Maybe a reasonable ROI on a bank robber, but not your standard Heather Reynolds.
FACIAL REC HAS IMPROVED
So this podcast episode probably couldn’t have existed ten, or even five years ago. We could have envisioned a future where police used facial recognition, but it just wasn’t practical enough at the time.
In the past few years, that’s changed. According to tests from NIST, the National Institute of Standards and Technology, the best facial recognition algorithms have improved from around 8% failure rates in 2010 to around 0.3% by 2018. These numbers are highly dependent on the kinds of images you feed these algorithms, but still: 99.7% accuracy is quite good. And that was three whole years ago.
Thus we end up with Clearview AI, which can identify faces at angles, or even with partial coverings like sunglasses and face masks. Or it won’t need to identify a face, because it can identify a tattoo instead. It’s a very powerful tool that, in the hands of law enforcement today, is having a real effect.
Which brings up the question: now that police can use facial recognition to catch criminals…should they?
BENEFITS
There are certainly arguments to the upside. For one thing, while facial recognition can’t catch a criminal on its own, it can certainly go a long way to aiding an investigation. Heather Reynolds is evidence to the point.
Plenty of Clermont residents were thrilled with how her case went down. On a police department Facebook post, Floridians lambasted her and her partner and celebrated their arrest. One woman posted an article highlighting the use of facial recognition in Reynolds’ capture: “She was caught; haven’t finished reading the article. But thank God she was caught!!” If you’re like this woman, the drawbacks to facial recognition are irrelevant in the effort to clean up crime in your town.
You may not feel as strongly about petty theft as this woman does. But what about if we were talking about murder, or terrorism? We all have a line beyond which the potential dangers of a technology are offset by their necessity. Take, for example, something that happened just recently.
“[Ted] when groups of people stormed the Capitol Building and now we know killing one police officer and wounding many others. You know, there was a crowd that essentially there weren’t enough police to handle. […] these folks all took pictures of themselves and each other and put them online and so in – where we have a crowd that is violent and committing crimes, I believe the use of facial recognition software is absolutely an important tool for the police to be able to have at their disposal.”
In these past few weeks, facial recognition has proved vital in identifying those captured on camera in the riot. The FBI alone collected over 100,000 images, which they’ve been analyzing ever since. Federal and state agencies have collaborated in fusion centers, trading information and images to determine which of their particular states’ residents were among the rioters. According to Hoan Ton-That, Clearview AI’s search traffic spiked 26 percent in the week following January 6th.
Isn’t at least some part of you glad that Clearview AI, shady as it is, exists right now? That dozens and possibly, by the end of all this, hundreds of rioters will be prosecuted because we have the tools to pick them out from a crowd?
Beyond the dramatic, high-stakes cases like this, there are also unemotional, practical reasons why facial recognition in law enforcement is a good thing. Like, here’s something you might not have considered: as precarious as it is to have a computer to identify criminals, humans are OGs of misidentification. There’s ample evidence that people make terrible eyewitnesses, because of our penchant for misremembering or outright creating false memories. As just one example: when The Innocence Project tracked 239 criminal convictions overturned by DNA evidence between 1990 and 2010, they found that three of every four of those cases had rested on eyewitness testimony. Put simply: when people are wrongly jailed, it’s usually because of other people misremembering things. That, of course, is not a problem machines have.
And here’s something else algorithms do better than us: recognizing faces. It’s crazy, but under the right conditions, the best facial recognition outperforms humans. They can spot little differences that our brains aren’t attuned to. This isn’t even news anymore. You can find studies going back seven, eight years where machines reliably outperform humans when the images are clear and head-on. Angles, blurriness and partial coverings are still problematic for them, but Clearview demonstrates how we’re improving there, too.
Considering all this, it only makes sense that facial recognition would be incorporated into law enforcement. It’s useful in catching baddies and, when used responsibly, is more reliable than eyewitness testimony.
But do these benefits ultimately outweigh the costs?
R. WILLIAMS I: POLICE VIEW
441 West Canfield St, Detroit. Wednesday. 6:44 P.M.
One evening in October 2018, a heavyset African-American man entered the Shinola luxury goods store in midtown Detroit. Dressed in a black jacket, black pants, white sneakers and a St. Louis Cardinals cap, he stood in front of a display of expensive watches just a few meters from the front door. Arms at his sides, he opened his hands wide–like a claw machine getting ready to grab. He took five watches, exiting the store with 3,800 dollars worth of merchandise.
An investigator for a loss prevention firm reviewed security footage, then handed it to Detroit police. Five months later, a digital examiner for the state police uploaded a still of the man to Michigan’s statewide facial recognition system.
Michigan’s system–the “Statewide Network of Agency Photos,” or “SNAP” for short–is a lot like Florida’s FACES. It too has a terrible name and a catchy acronym. It too costs a whole ton of money: 5.5 million dollars for a 7-year contract with the state that began in 2014. It too uses mugshots, driver’s licenses and other photos of its state’s citizens, in a database totalling 50 million images. For context, less than 10 million people live in Michigan.
SNAP’s unique characteristic is that it’s built on two, separate algorithms: one developed by a small Colorado company called Rank One, and the other by NEC, a Japanese multinational corporation. In practice what this means is that, when that digital examiner uploaded Shinola’s surveillance photos to SNAP, it returned two sets of results–one from each algorithm.
The examiner passed on the results to Detroit police. According to the New York Times, the police used it to put together a photo lineup for the loss prevention investigator–the woman from earlier who was first to see the security footage. They asked her to point out the culprit, and she did.
On a Thursday afternoon in January, 2020–over a year after the theft took place–Detroit police phoned a man named Robert Williams. He was at work. They asked him to come into the station and turn himself in for arrest. He didn’t take their request seriously.
Later that day police intercepted Williams at his home. They arrested him, and took him to jail.
The following day, around noon, they brought him into an interrogation room. They laid three pieces of paper, face down, on the table.
“When’s the last time you went to a Shinola store?” one of the detectives asked.
He told them he’d visited with his wife in 2014, when it first opened.
The detective flipped over the papers–the surveillance stills from Shinola. “Is that you?”
One of the images was a close-up–a bit blurry, but clear enough to make out the shoplifter’s face. Robert Williams took the paper, and held it up to his face.
“No, this is not me. You think all black men look alike?”
From the New York Times, quote:
“In Mr. Williams’s recollection, after he held the surveillance video still next to his face, the two detectives leaned back in their chairs and looked at one another. One detective, seeming chagrined, said to his partner:
“I guess the computer got it wrong.””
R. WILLIAMS II: HIS VIEW
Robert Williams did visit the Shinola store back when it opened in 2014, with his wife. At the time of the 2018 robbery, though, he was driving home from work. We know this because he posted an Instagram video from his car, singing along to the radio.
But why would an innocent man hang up on the cops when they called his office a year later, saying he was to be arrested? Because he thought it was a prank call. Obviously! He was sitting at his desk on an ordinary work day. If somebody did the same thing to you, you’d probably think they were a scam artist.
So when Robert left work and drove to his quiet, suburban home that evening in January, 2020, he had no concept that anything bad was about to happen. It only hit when he pulled into his driveway, and a cop car pulled up behind him, blocking the path back out.
It must’ve been pretty scary. Robert got out of his car, and so did the police officers. According to the New York Times, which broke the story, they grabbed and cuffed him but wouldn’t say why he was being arrested. Instead, they showed him a piece of paper with his photograph on it, along with the words “felony warrant” and “larceny.”
As this was unfolding, Robert’s wife and two young daughters ran out onto the front lawn. The girls–one five years old, the other even younger–reacted as you’d expect them to. Ms. Williams asked the cops where they were taking her husband.
“Google it,” one of them replied.
You could imagine the embarrassment, the fear and the anger. And whatever mix of emotions Robert might have felt when, after spending a night in jail, he was shown surveillance images of a man who was not him. From the Times report, quote:
“Mr. Williams was kept in custody until that evening, 30 hours after being arrested, and released on a $1,000 personal bond. He waited outside in the rain for 30 minutes until his wife could pick him up. When he got home at 10 p.m., his five-year-old daughter was still awake. She said she was waiting for him because he had said, while being arrested, that he’d be right back.”
That day Robert spent in jail was the first time he’d missed a day of work in four years.
MISMANAGEMENT
There’s a saying: to err is human, but to really screw things up, you need a computer.
Facial recognition, on its own, did not arrest Robert Williams. But his story is a perfect case study in how facial recognition, improperly handled, can really screw things up.
Let’s start with the initial facial recognition query. When the examiner sent the results to police, it came in the form of an “investigative lead report.” The very top of the document contains a warning in bold and all-caps font. Quote:
“THIS DOCUMENT IS NOT A POSITIVE IDENTIFICATION. IT IS AN INVESTIGATIVE LEAD ONLY AND IS NOT PROBABLE CAUSE TO ARREST. FURTHER INVESTIGATION IS NEEDED TO DEVELOP PROBABLE CAUSE TO ARREST.”
End quote. You don’t need to be a trained police officer to figure out what that means. The SNAP algorithm yielded multiple possible suspects, none with 100% confidence. It was interpreting grainy photographs that didn’t even show a clear image of the subject’s face. Therefore, further investigation would be needed.
But what kind of investigating did the cops do after receiving those results? They didn’t gather any evidence on Robert Williams, or check to see whether he had an alibi, like a singalong video posted to his Instagram while the robbery was taking place.
The only further investigating they did was to reach back out to the loss prevention agent who’d originally passed on the surveillance footage to them. According to the Times, they provided her with a lineup of six photographs. Then they asked her to pick out the culprit. She picked Robert.
But was this woman qualified to make such a call? For one thing, she wasn’t an eyewitness to the incident, only the surveillance footage. So, really, she was no more qualified to identify the culprit than anyone else who looked at those same photos. And even if she were at the scene that day, by the time she was shown the lineup, it was already half a year later. How long can you remember a stranger’s face?
EXPLANATIONS
There’s no question that Robert Williams was wronged, and the police involved could have done more work before jumping to conclusions. But there are a few different ways of explaining how an error of such proportions could have occurred.
You could, of course, simply say that those cops are bad at their jobs. But this is obvious, and it really doesn’t get us anywhere. The systemic mismanagement that occurred at multiple levels of the investigation–from the investigators to the loss prevention agent, right down to SNAP itself–suggests a deeper problem.
Here’s one way of looking at this story: facial recognition is too accurate for its own good.
Yes, sure, that sounds like the exact wrong takeaway here. But you see, back when facial recognition just wasn’t that great, police didn’t put too much stock in it. In Florida, FACES only led to a couple thousand arrests in two decades. Another system deployed in Santa Ana, California, led to just one arrest in four years. The cops never relied on this tech, because it wasn’t effective.
Now that the tech is good, it’s being relied on more. And that’s a problem. Yossi Naar, CVO at Cybereason:
“[Yossi] The possibility of mistaken identification is well-ingrained in our understanding of the world and in our understanding of ourselves and other humans and we can apply that. When it comes to computers, we tend to think about them as infallible things and it’s very, very easy to forget that computers are as fallible as the people that create them. The more kind of magic that we see in the way that they operate, the less we remember that they can be just as wrong.
So when the mistakes are very obvious and grotesque, it’s easy to say, “Oh, it’s just a computer. It made a mistake.” But when you kind of tend to trust the system and assuming that in this case that we’re using a system that they’ve used and they know that it kind of works, it works pretty well, it gives good matches. If it identified the right person 10 percent of the time, they would know not to trust it and perhaps stop using it.
But if it identifies the right person 99 percent of the time, so now you have a case where the system tells you that this is the person. The person is telling you it’s not me. It’s somebody else. Your eyes maybe even tell you it kind of looks like somebody else. But you don’t trust your eyes because you understand that there’s a 90 percent probability that the computer is right.
You just trust it more than you trust your own judgment.”
This is the kind of thing we mean when we talk about issues with facial recognition in policing. You don’t need to go as far as China, or 1984–it can be as simple as cops not being qualified to use such a complex technology. The SNAP algorithm yielded two sets of half a dozen possible matches based on poor images captured by surveillance cameras, so we can reasonably assume that the quality of its results weren’t perfect. The cops–either because they didn’t know better, didn’t care to look into it, or didn’t want to disagree with the computer–interpreted the output wrongfully, creating a lineup that probably never should have existed for an investigator definitely not qualified to make a verdict on it. The one qualified person in this whole chain of causality who understood the tech–the person who prepared the initial report on SNAP’s results–predicted these dangers, and tried to send a clear warning. It didn’t work.
So handing facial recognition to these officers was a risky idea. A bit like if you handed an assault rifle to a dorky AI programmer–the gun can’t hurt anyone on its own, sure, but that nerd probably doesn’t know how to turn on the safety, so you damn well don’t want to be in the room when he picks it up.
RACIAL BIAS
So that’s one interpretation of what might have gone wrong. Of course, we also have to address the elephant in the room.
Robert Williams is a big black guy. In general, people who look like him get a bad deal in the American law enforcement system. Would the Detroit police have handled things differently if he were white? Would they have spent more time gathering evidence, or looked more closely at that photograph before jumping to conclusions? There is certainly an argument to be made.
But the racial influence in this story goes even deeper than this. Because right from the beginning, the system was stacked against Robert.
“[Ted] The way that it works is that facial recognition programs tend to be better at recognizing, identifying and labeling white men than it is anybody else.”
In 2019, NIST conducted a study of 189 facial recognition algorithms out on the market, to see how demographics impacted their accuracy. They tested these algorithms on 18.27 million images of people of different age, sex and race. The results were crazy bad. They found that the algorithms misidentified people of certain demographic groups 10 to 100 times more often than middle-aged white men. Across the board, black and asian men yielded more false positives than white men. Women yielded more false positives than men across all ages and races. And Native Americans? Well, you might as well pour coffee on the computer.
“[Ted] If you are a person of color, it’s less likely frankly that the facial recognition program is going to identify you. However, it is more likely than if you were not a person of color that the facial identification program will misidentify you.”
As we mentioned earlier, Michigan’s SNAP system is based on two different algorithms. One of them–the one developed by Japanese company NEC–was not fully included in NIST’s study. The researchers did have high praise for it, but for reasons too complicated to get into here, they couldn’t evaluate it as they did the others.
The second algorithm SNAP relies on–the one developed by Colorado company Rank One–was thoroughly studied as part of the NIST test. Not only did it show racial biases in line with the trends the researchers found in other algorithms, it was also one of the worst performing algorithms in general out of all 189 tested.
HOW CAN AI BE BIASED?
“[Nate] Could you explain how AI can even have such biases in the first place? Because that’s not really an intuitive idea.
“[Yossi] Let’s think about how artificial intelligence is trained, right? [. . .] let’s take a thing that’s not what we’re talking about just to kind of simplify the example.
I want to be able to identify what a can of soda looks like, right? So I’m going to take a bunch of cans of soda and I’m going to photograph them in different ways. Maybe I’m going to run a Google search and search for a can of Coke or can of soda, take all of these images and then this is my database of confirmed stuff.
Then I’m going to take a database of stuff that’s not kinds of soda. Cats, people, cars. I don’t know, scenery. And I’m going to train my algorithm to kind of – I’m going to give it positive reinforcement in a sense whenever it correctly identifies a can of soda and I’m going to give it negative reinforcement when it identifies a thing that’s not that.”
That saying “you are what you eat” is much truer of computers than it is of humans. An algorithm really is only as good or bad, or as biased, as the data you feed it.
“[Yossi] the bias really starts at the engineering level unfortunately. [. . .] So they’re going to take whatever data they have easy access to. So if there’s a lot more Caucasian people that have a large collection of photos of them, maybe I would use PR photos of people. Maybe I would use publicly available databases or people that have signed up for this particular data collection task.
I’m going to get more photos of Caucasians. Then I’m going to get of other minorities. Unless I specifically try not to, right?”
There are all kinds of little reasons why facial recognition performs worse among certain groups, from makeup to color contrast in skin tones and biases in cameras themselves.
“[Ted] some bias is camera bias. [. . .] So if somebody is in shade and their skin is particularly dark, then the camera may not be able to make those distinctions as well.”
And it may be that programmers–who, let’s face it, don’t spread equally across the demographic spectrum–unwittingly apply their own biases when training their software.
But the biggest reason for racial bias in algorithms is training data. There are more pictures of certain kinds of people than others in the places where programmers draw from. Native Americans are perfect evidence to the point: there just aren’t as many images of Native Americans floating around as there are of white people, so the algorithms suffer.
So as much as facial recognition is, indeed, getting better by the year, it’s also getting better at disproportionate rates for different kinds of people. African-Americans are already disproportionately targeted by law enforcement as a result of human biases, so it’s a double whammy that this technology is becoming more widespread.
FIXING BIASES
Perhaps police use of facial recognition would be less controversial if these racial biases weren’t an issue. So let’s talk about how it can be fixed.
The first, most obvious fix is to simply use better datasets–to consciously track whether your training data accounts for all demographic groups, and test these algorithms for bias before they make it onto the market.
Let’s say that’s not an option: that the data available to you is unequal, and there’s no way to fix it. Another potential means of mitigating bias is to weigh your input data, in such a way that compensates for any discrepancies in volume. It can get pretty complicated so, as an analogy, imagine you’re taking a survey.
Say you’re polling a town of 1,000 people about who they want to be mayor. Half the town is over the age of 50, half under. Young people hate surveys, so most of the 100 people who told you who they’re voting for are old. In that case, you might give more weight to the young people who responded to your poll, in order to approximate a more accurate representation of the population. Of course, young people might be less likely to vote, and some of them can’t vote at all. So you can see how weighting data is both important, and very complicated.
Weighting data is common in AI when you’re dealing with different kinds of data that come in different volumes, or are more or less important than one another. In facial recognition it’s tough–you can’t exactly say that one Native American face is worth two white faces, to make your results more accurate. But even if weighting data can’t fix accuracy, it can fix presentation.
Just about every facial recognition program uses some form of “confidence scores” to rank the accuracy of its results. SNAP, for example, would’ve given Robert Williams’ picture a confidence score–95%, 80%, 50%–indicating how much the Shinola surveillance footage aligned with his face. With data weighting, a program like this could be made to give lower confidence scores for demographic groups it has trouble with. It won’t fix the inaccuracy of the software, but it might give the humans reading these results more pause before they jump to conclusions.
Perhaps that’s a really minor fix for a really big problem. But don’t worry: we’ve saved the best solution for last.
As we mentioned, the way to make facial recognition algorithms accurate is to use good input data. But making sure your input data is sufficient across demographic groups is tough. That is, unless you indiscriminately take billions of photos from around the web–so many photos that there’s no way any kind of person in the world isn’t entirely accounted for.
Do you see where this is going?
CLEARVIEW’S BIAS SOLUTION
In October 2019, Clearview AI commissioned an independent study as to the accuracy of its program across demographic groups. It was a problematic study, but also incidentally insightful.
Clearview’s study was modeled after a different study published one year earlier, by the ACLU, which found that Amazon’s “Rekognition” software–that’s “recognition” but with a “k”–in testing, matched dozens of congress people with criminal mugshots. The congresspeople it misidentified included plenty of white men, but skewed African-American and Latino.
Clearview’s study was clearly a shot at Amazon: trying to prove that under the same conditions, their software would be more accurate and less biased. And indeed, in the final report, the examiners wrote that, quote:
“The Independent Review Panel determined that Clearview rated 100% accurate, producing instant and accurate matches for every photo image in the test. Accuracy was consistent across all racial and demographic groups.”
Now, that result–100%–seems phishy. And it is. But not for the reasons you might suspect.
Clearview didn’t manipulate the methodology of the test, or the final result. It really did come back 100%. The reason their algorithm was perfect, though, is because Clearview has dozens, even hundreds of pictures of every U.S. congressperson. They take images from all over the internet, so unlike Rekognition–for which each picture was new data–Clearview might have already had the pictures it was being tested on in its database. If not, it had dozens others like it.
This, obviously, is unfair. We don’t know how Clearview would perform with pictures not yet on the web, or of people who aren’t famous. But in a way, that 100% result isn’t entirely phony. Clearview is almost certainly more accurate and less racially biased than Rekognition, simply by virtue of just how many photos it has of people of every race, color and creed. Clearview AI may not have a bias problem–or at least, as bad a bias problem–as its competition, because its reference data is so massive and diverse. When you steal images from every corner of the internet, you’ll even end up with enough Native Americans.
So is Clearview AI a problem, or the solution? Today, police across North America are using more accurate, less biased software. But that road is paved with serious issues about privacy and data theft.
Where you fall on this question is probably the same as where you fell on Heather Reynolds, the ACE Hardware bandit. The residents of Clermont who celebrated Heather’s capture didn’t appear to give any thought to her personal privacy. The less sympathetic you are to the criminal who’s caught, the more likely you are to justify the means by which they’re apprehended. But if Heather’s story rubbed you the wrong way–you didn’t like the use of facial recognition, and how Clearview used her Facebook pictures–then you probably don’t care how accurate and unbiased Clearview may or may not be. It’s creepy, and a dangerous precedent to set.
But the dangers go way past Heather Reynolds, or even Robert Williams. Last summer citizens took to the streets in cities across America to protest police violence and, in many cases, were filmed doing it. That footage is in law enforcement databases right now, waiting.
Our next Malicious Life episode is about what happens when you combine police, protestors and facial recognition. The results of that mixture can change lives, for better and for worse.